Unlocking Business Potential with Bayesian Network Analysis
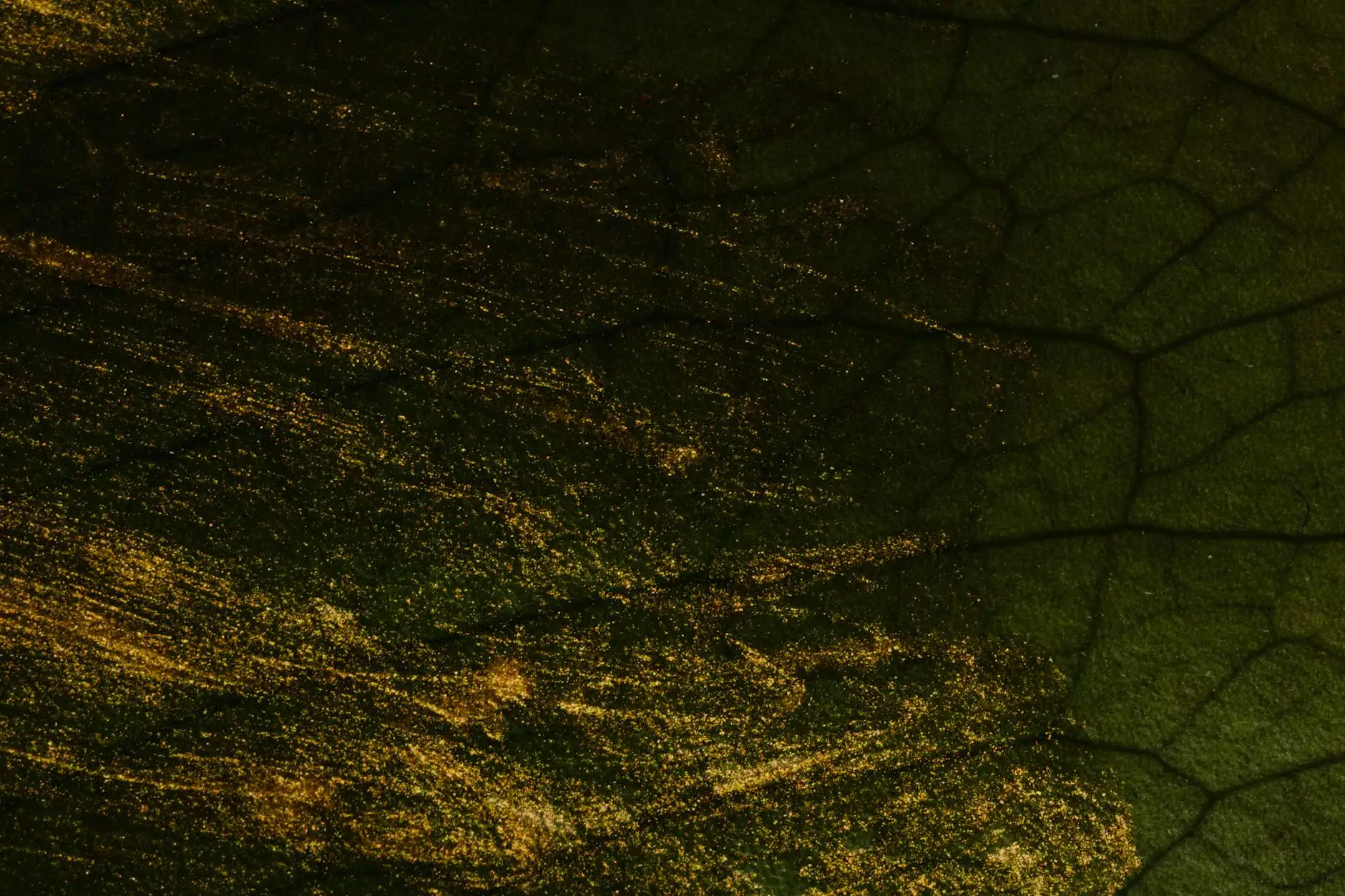
In today's rapidly evolving business landscape, companies are constantly seeking innovative methods to enhance their decision-making processes and optimize performance. One such method that has gained significant traction is Bayesian Network Analysis. This powerful statistical tool offers a robust framework for modeling complex relationships within data, enabling businesses to make informed decisions that drive growth and innovation.
Understanding Bayesian Network Analysis
Bayesian Network Analysis is a probabilistic graphical model that represents a set of variables and their conditional dependencies using directed acyclic graphs (DAGs). This statistical framework allows for the mapping of complex relationships, making it especially useful for sectors like IT services and security systems, where data-driven decision-making is crucial.
The Core Components of Bayesian Networks
To fully appreciate the impact of Bayesian Network Analysis in business, it is essential to understand its core components:
- Nodes: Each node in a Bayesian network represents a random variable, which can be either observable data or latent (hidden) factors.
- Edges: The directed edges connecting the nodes illustrate the relationships between the variables, indicating how a change in one variable may affect another.
- Conditional Probability Tables (CPTs): These tables define the probability distributions of each node given its parent nodes, quantifying the strength of the relationships in the network.
Applications of Bayesian Network Analysis in Business
Bayesian Network Analysis is revolutionizing how businesses operate in various domains, specifically in IT services and security systems. Here are some of the most impactful applications:
1. Risk Assessment and Management
In the realm of security systems, evaluating potential threats and vulnerabilities is critical. Bayesian networks can model the probabilities of different risks and their potentially devastating impacts. This context allows companies to:
- Identify high-risk areas in their IT infrastructure.
- Quantify the potential costs associated with various security breaches.
- Prioritize their investment in security measures based on the most probable risks.
2. Improving Customer Insights
Understanding customer behavior is crucial for any business. Bayesian networks can analyze complex customer data, helping companies detect patterns and trends that inform marketing strategies. By leveraging this analysis, businesses can:
- Segment their target markets more effectively.
- Predict customer needs and preferences based on historical data.
- Personalize offers to improve customer engagement and retention.
3. Enhanced Decision Making
When faced with uncertainty, traditional decision-making processes can lead to suboptimal outcomes. Bayesian networks provide a structured approach to decision-making under uncertainty, allowing businesses to:
- Incorporate expert opinions and empirical data into their decision matrices.
- Simulate various scenarios to evaluate possible outcomes before committing to a course of action.
- Make more informed strategic decisions that align with their long-term goals.
Building a Bayesian Network: Step-by-Step Guide
Creating a Bayesian network can initially seem daunting, but by following a systematic approach, businesses can develop a model tailored to their specific needs. Here is a comprehensive guide:
Step 1: Define the Problem
Start by clearly defining the business problem you aim to solve. This step will guide the structure and development of your Bayesian network. Identify the key variables that influence the problem.
Step 2: Gather Data
Data collection is crucial for building a Bayesian network. Collect both quantitative and qualitative data relevant to the identified variables. This may involve:
- Surveys or questionnaires for customer insights.
- Historical performance data from internal systems.
- Market research reports and industry analyses.
Step 3: Construct the Network
Map out the relationships among the variables using a directed acyclic graph. This network visually represents how each variable interacts with one another, which is fundamental for understanding dependencies. Use software tools like Netica, GeNIe, or Bayes Server for this task.
Step 4: Specify Conditional Probability Tables
Assign probabilities to the relationships in your network using your collected data. This step involves estimating the conditional probabilities based on historical data or through expert judgment.
Step 5: Validate the Model
Once the network is constructed, validate it by testing its predictions against real-world outcomes. Adjust the model as necessary based on feedback and new data.
Step 6: Implement and Monitor
Integrate the Bayesian network into your decision-making processes. Continuously monitor the outcomes and refine the model based on real-time data and feedback from stakeholders.
Benefits of Implementing Bayesian Network Analysis
Incorporating Bayesian Network Analysis into business operations provides numerous advantages:
- Enhanced Predictive Accuracy: Bayesian networks can improve the accuracy of predictions by considering various possible influences and dependencies among data points.
- Flexibility and Adaptability: As new data becomes available, Bayesian networks can be updated seamlessly, allowing businesses to adapt to changing environments efficiently.
- Cost-Effective Decision-Making: By simulating different scenarios, businesses can save resources by identifying the most effective course of action before implementation.
- Better Communication of Uncertainty: Bayesian networks effectively communicate uncertainties within the decision-making process, allowing stakeholders to understand and accept risks more effectively.
Real-World Success Stories
Many businesses have successfully harnessed Bayesian Network Analysis to transform their operations:
Case Study: IT Service Provider
An IT service provider implemented a Bayesian network to optimize its resource allocation and service management. By analyzing historical data and real-time incidents, they were able to:
- Reduce downtime by 30% through proactive management of resources.
- Enhance customer satisfaction due to improved service response times.
Case Study: Security Systems Company
A leading security systems company adopted Bayesian analysis to assess threat levels dynamically. By continuously analyzing data from various sources, they improved their risk assessment process, resulting in:
- A 50% reduction in response times to potential security threats.
- Increased client trust due to enhanced security measures.
Challenges to Consider
While Bayesian Network Analysis offers extensive benefits, there are challenges that businesses must navigate:
- Data Quality: The accuracy of a Bayesian network is highly dependent on the quality of the input data. Ensuring data integrity is paramount.
- Complexity: Constructing and interpreting Bayesian networks can be complex, requiring specialized knowledge and skills from staff.
- Time Investment: Building an effective model requires significant time and resources, especially in the data collection and validation phases.
Future Trends in Bayesian Network Analysis
The future of Bayesian Network Analysis in business looks promising, with several emerging trends on the horizon:
- Integration with Machine Learning: Combining Bayesian networks with machine learning techniques to enhance predictive capabilities.
- Real-Time Data Processing: Using big data analytics to allow for real-time updates and adaptive models.
- Automated Bayesian Network Construction: Emerging tools that automatically construct Bayesian networks from data, reducing the manual effort involved.
Conclusion
Bayesian Network Analysis is a powerful tool that can significantly enhance decision-making processes in the business environment. By leveraging its capabilities, companies in IT services and security systems can unlock new opportunities, mitigate risks, and drive sustained growth. Investing in this analytical framework not only improves operational efficiency but also positions businesses to adapt and thrive in an increasingly complex marketplace.
For organizations looking to leverage the full potential of Bayesian networks, it's crucial to foster a culture of data-driven decision-making, continuously enhance data collection methodologies, and invest in the necessary training for staff. In doing so, they can ensure that they not only stay competitive but also lead in their respective industries.