Unlocking Business Potential with Machine Learning Data Annotation
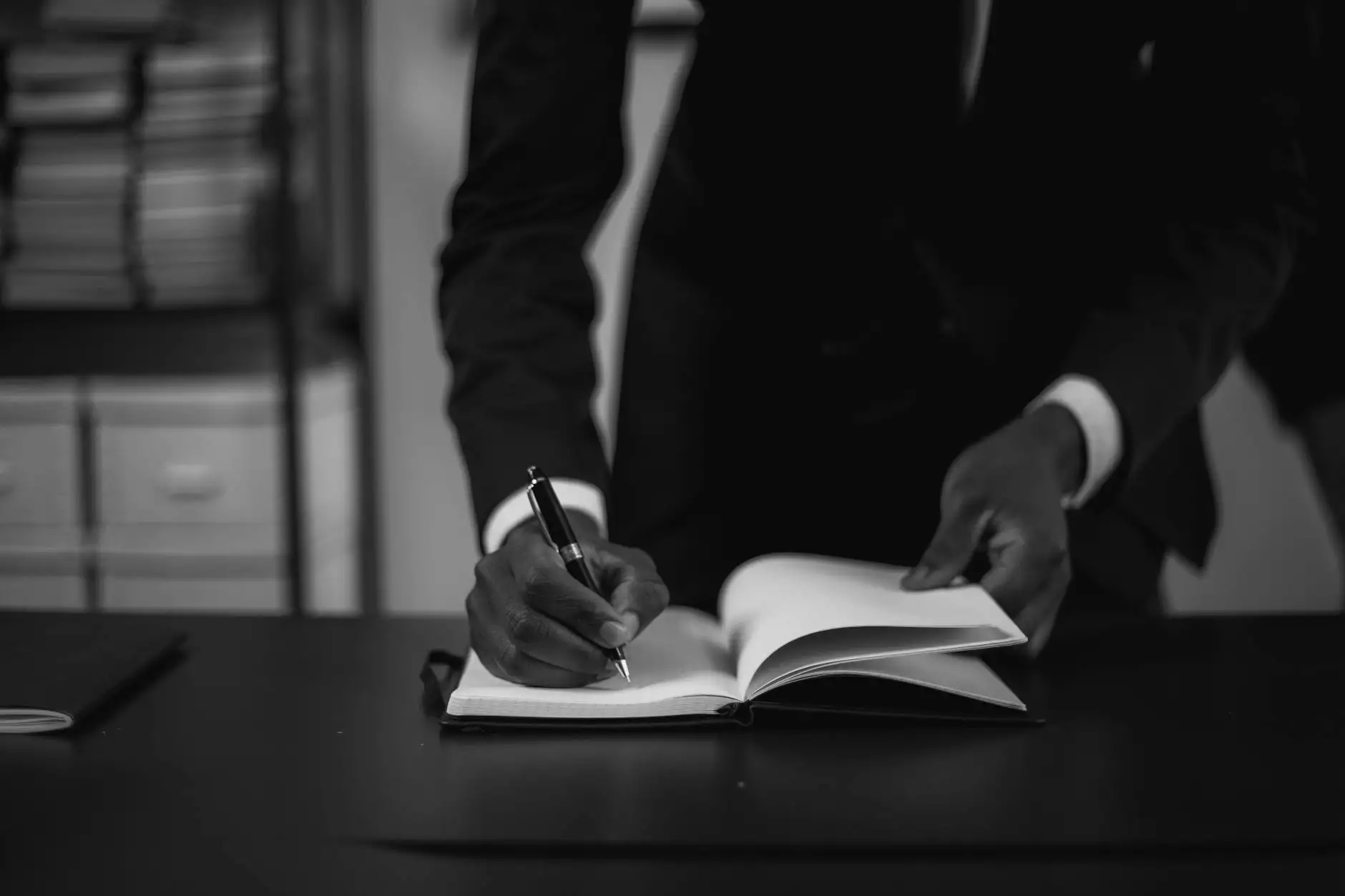
In today’s rapidly evolving technological landscape, machine learning data annotation plays a pivotal role in facilitating advancements in artificial intelligence (AI). For businesses looking to harness the power of AI, understanding the significance of data annotation is crucial. This article delves into how machine learning data annotation can elevate your business strategies, especially within the realm of software development.
What is Machine Learning Data Annotation?
Machine learning data annotation is the process of labeling datasets to enable machine learning algorithms to learn and make predictions. This intricate process involves categorizing various types of data—such as images, text, and videos—into comprehensible formats that machines can interpret. As the backbone of AI functionalities, data annotation ensures that machine learning models are trained on high-quality, accurately labeled data.
The Importance of Machine Learning Data Annotation in Business
As companies pivot towards data-driven decision-making, the relevance of robust data annotation cannot be overstated. Here’s why:
1. Enhancing Model Accuracy
High-quality data directly correlates to the effectiveness of machine learning models. The more precise the data annotation, the more accurately a model can predict outcomes. Businesses leveraging machine learning data annotation can expect:
- Improved predictive analytics
- Enhanced customer targeting
- Increased operational efficiency
2. Accelerating Development Cycle
With accurate data annotation, software developers can significantly reduce the time spent in model training. Rapid iteration of models allows for:
- Faster deployment of machine learning applications
- Quicker adaptation to market changes
- More responsive customer service solutions
3. Informing Business Strategies
The insights derived from properly annotated data can inform vital business strategies. Companies can utilize these insights to:
- Understand customer behavior
- Identify market trends
- Refine product development processes
Types of Data Annotation Techniques
Understanding the various types of data annotation techniques is essential for businesses aiming to implement effective machine learning solutions. Here are some prominent techniques:
1. Image Annotation
This involves labeling images, typically for computer vision tasks, using bounding boxes, polygons, or segmentation masks. Businesses in sectors like e-commerce and security use image annotation to train models for object detection, facial recognition, and more.
2. Text Annotation
Text annotation includes identifying and labeling entities, sentiment, and intent in textual data. This is essential in natural language processing applications, such as chatbots and sentiment analysis. Businesses can enhance customer interaction through effectively annotated text data.
3. Video Annotation
Video annotation is crucial for developing systems that require interaction with real-time video feeds. This technique plays a significant role in sectors such as autonomous driving, security surveillance, and sports analysis.
Best Practices for Effective Data Annotation
To ensure the success of machine learning data annotation efforts, businesses should adhere to the following best practices:
1. Define Clear Annotation Guidelines
Providing clear and comprehensive guidelines for annotators can greatly enhance the quality of the annotation process. Guidelines should include:
- Specific examples of labeled data
- Common pitfalls and how to avoid them
- Criteria for different annotation types
2. Utilize Quality Control Measures
Incorporating quality control checks—such as adding redundant labeling and regular audits—ensures that the annotation data remains accurate and reliable. Businesses can implement:
- Cross-validation of labeled data
- Feedback mechanisms for annotators
- Random sampling of annotated datasets for verification
3. Invest in Training and Tools
Investing in the right tools and providing regular training to annotators enhances the efficiency and accuracy of the annotation process. This includes:
- Leveraging AI-assisted annotation tools
- Conducting workshops for annotators
- Utilizing platforms for collaborative annotation efforts
Choosing the Right Data Annotation Partner
For businesses without the in-house resources for data annotation, selecting the right partner is essential. Consider the following factors:
1. Expertise and Experience
Choose data annotation partners with a proven track record in your specific industry. Their experience will often equate to higher quality output, a critical factor for successful machine learning projects.
2. Customization and Scalability
Look for partners who offer customizable solutions and can scale their services based on your evolving needs. This adaptability is crucial for long-term business success.
3. Technology Integration
Ensure that the data annotation partner can seamlessly integrate their operations with your existing technological infrastructure, enhancing collaboration and efficiency.
Case Studies: Success Stories with Machine Learning Data Annotation
Real-life examples can illuminate the tangible benefits of machine learning data annotation:
1. E-commerce Enhancement
An online retail giant implemented machine learning data annotation to improve its product recommendation system. By accurately annotating user behavior data, the company achieved:
- A 25% increase in click-through rates
- A significant uplift in customer retention
- Higher sales volume from personalized marketing
2. Autonomous Vehicle Development
A leading automotive manufacturer used machine learning data annotation to train its self-driving vehicle systems. Through comprehensive video and image annotation, they:
- Enhanced object recognition capabilities
- Reduced incident response time by 40%
- Achieved regulatory compliance with safety standards
The Future of Machine Learning Data Annotation
The landscape of machine learning data annotation is continually evolving. Emerging technologies such as automated annotation, AI-driven tools, and improved algorithms promise to enhance efficiency and effectiveness. Businesses that stay ahead of these trends are poised to benefit from:
- Increased accuracy and speed in data processing
- Reduction in operational costs associated with manual annotation
- Enhanced capabilities for tackling complex challenges across industries
Conclusion
As businesses increasingly rely on machine learning technologies, the significance of machine learning data annotation becomes undeniable. By investing in quality data annotation processes, businesses can unlock new potentials, enhance software development efforts, and ultimately drive significant growth. Whether you are a startup or an established enterprise, focusing on data annotation can position your business at the forefront of the AI revolution.
For companies looking to embark on this transformative journey, partnering with experts in machine learning data annotation is vital. At Keymakr, we specialize in providing high-quality data annotation services that cater to the unique needs of your business, ensuring that you harness the full potential of machine learning. Reach out to us today to learn more about how we can help you achieve your business objectives!